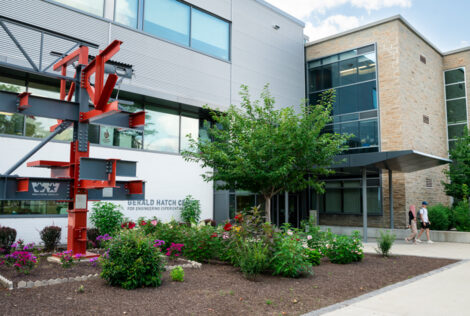
Expertise
Areas of Specialization
Research Clusters
-
Professor Emeritus
Electrical & Computer Engineering
-
Professor Emeritus
McMaster School of Biomedical Engineering
Overview
Dr. Reilly works at the interface of machine learning and signal processing applied to health related problems, particularly in neuroscience and psychiatry. Particular thrusts are development of improved machine learning algorithms, diagnosis and treatment of psychiatric illness, prognosis for coma outcome, and assessment of infant motor movement relating to neurological deficit.
Did you know?
Dr. Reilly was the Acting Chair in 2011, Associate Chair Undergraduate Affairs 2004 – 2013 and is a holder of 9 patents
Dr. Reilly and colleagues received the R.O. Jones Best Paper Award for their presentation at the Canadian Psychiatric Association Annual Conference, Sept. 2014, Toronto.
Professor James Reilly graduated with a B.A.Sc. degree from the University of Waterloo in electrical engineering in 1973. He received his M.Eng and Ph.D. from McMaster University in 1977 and 1980 respectively, both in electrical engineering. He worked from 1973-75 in the cable TV industry, and from 1980-85 at then Bell-Northern Research in Ottawa, where he was involved in the development of terrestrial digital microwave radio and multiplexing technology for digital transmission systems. He joined the faculty of McMaster University in 1985 as an associate professor, and was promoted to the rank of full professor in 1992. He is an electrical/biomedical engineer at McMaster University, specializing in signal processing and machine learning. He has published widely in the area of signal processing at large and has co-authored pioneering papers on machine learning analysis of the EEG for the treatment of major depression and schizophrenia. He is a member of the IEEE MLSP Technical Affairs Committee and has enjoyed extensive collaboration and funding from industry, through which he has obtained 9 patents.
B.A.Sc. (Waterloo, Canada) ; M.Eng., Ph.D. (McMaster, Canada)
Dean’s Honour Role for Excellence in Teaching (awarded an estimated 7 times)
Selected
Ahmad Khodayari-Rostamabad, Gary M. Hasey, Duncan J. MacCrimmon, James P. Reilly and Hubert de Bruin (2010)
Machine Learning Methodologies Using Pre-Treatment Electroencephalography Can Predict The Symptomatic Response To Clozapine Therapy
CLINICAL NEUROPHYSIOLOGY, VOL. 121, ISSUE 12, DEC. 2010, PP. 1998 – 2006.
This article is one of the first articles to appear on the use of machine learning methods for assessing treatment for psychiatric illness.
A. Khodayari-Rostamabad, J.P. Reilly, G.M. Hasey, H. de Bruin and D.J. MacCrimmon (2013)
A Machine Learning Approach Using EEG Data to Predict Response to SSRI Treatment for Major Depressive Disorder
CLINICAL NEUROPHYSIOLOGY, VOL. 124, NO. 10, OCT. 2013, PP. 1975 – 1985.
This is a pioneering article showing how analysis of the EEG using machine learning methods is useful for treating major depression.
Recent
Maryam Ravan, Duncan MacCrimmon, James P. Reilly, Gary Hasey, and Ahmad Khodayari-Rostamabad, “A Machine Learning Approach Using Brain Source Localization on P300 Responses to Investigate the Effect of Clozapine Therapy”, Clinical Neurophysiology Vol. 126, Issue 4, April 2015, pp.721 – 730.
Narges Armanfard, James P. Reilly, Majid Komeili, “Local Feature Selection for Data Classification”, IEEE Trans. Pattern Analysis and Machine Intelligence, Vol. 38, No. 6, June 2016, pp. 1217-1227.
Nasser Mourad, James P. Reilly, T. Kirubarajan, “Majorization–Minimization for Blind Source Separation of Sparse Sources”, Signal Processing, Vol 131, Feb. 2017, pp. 120 – 133.
Amabilis Harrison, Michael Noseworthy, James Reilly, Weiguang Guan, John Connelly, “EEG and fMRI agree: mental arithmetic is the easiest form of imagery to detect”, Consciousness and Cognition, Vol. 48, Feb. 2017 pp. 104-116.
Narges Armanfard, James P. Reilly, Majid Komeili, “Feature Selection and Classification Based on Local Modeling of the Sample Space”, accepted by IEEE Trans. Neural Networks and Learning Systems, Feb. 2017. 1